#9 — The Uber Safety Report & Blind Spots in Product Design
A few weeks ago, Uber released its first ever US Safety Report analyzing data from 2017 and 2018. The statistic that grabbed headlines was that in 2018, over 3,000 cases of sexual assault had been reported on the app.
While issuing the report is a step in the right direction, Kara Swisher argues in There Is a Reason Tech Isn’t Safe that the root cause of Uber’s safety issues remain widespread across Silicon Valley:
“Simply put, far too many of the people who have designed the wondrous parts of the internet — thinking up cool new products to make our lives easier, distributing them across the globe and making fortunes doing so — have never felt unsafe a day in their lives.”
They’ve never felt a twinge of fear getting into a stranger’s car. They’ve never imagined the pain of privacy violations, because rarely have they been hacked or swatted or doxed. They’ve not been stalked or attacked or zeroed out because of their gender, race or sexual orientation. They’ve never had to think about the consequences of bad choices, because there have been almost no consequences of failure.”
Uber’s product and engineering teams are overwhelmingly male. According to The Verge, over 80% of the company’s engineers are male and more than 90% are either white or Asian. Personal safety typically isn’t a top of mind issue for most males. As a result, Uber’s engineering team had a blind spot and safety features were not a priority in the design process.
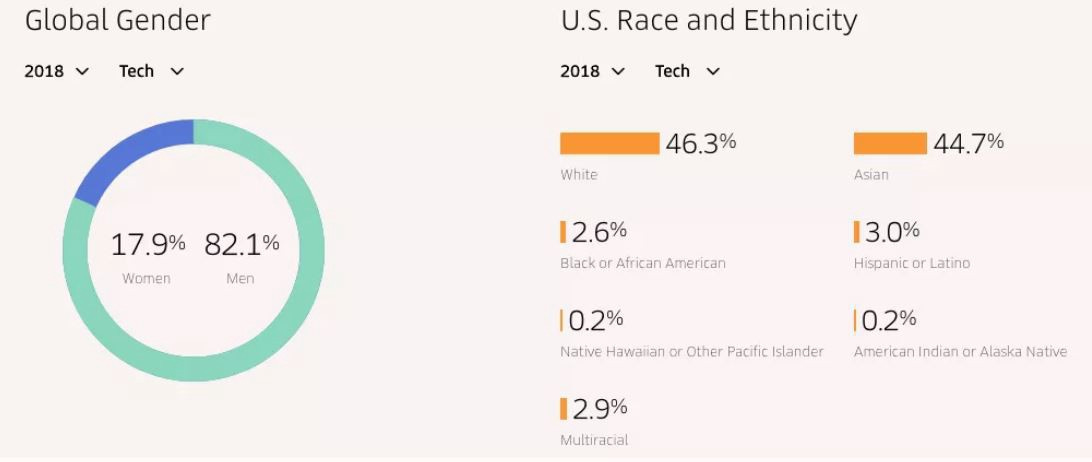
One of the most troubling aspects of Swisher’s argument is that this is not a bug:
“The real problem…is that thoughtlessness is a feature, lack of reflection is a feature, a drive to grow at all costs is a feature and, most of all, the sloppy and lazy ways in which tech too often designs and deploys its inventions are the ultimate features.”
Unfortunately, this issue is not isolated to Uber, a point made clear in Invisible Women: Data Bias in a World Designed for Men by Caroline Criado Perez. From the Financial Times review of the book:
“The book covers a huge range of examples of how data are biased against women — from industrial design to healthcare systems to disaster responses. As Criado Perez says, most, if not all, of these examples did not come about because men deliberately excluded women from the data, but because they just didn’t think about them.”
One example involves algorithmically screening resumes:
“Algorithmic scanning of CVs is a particularly problematic area. She cites the example of Gild, a tech hiring platform, which uses algorithms to analyse candidates’ online presence to identify the best computer programmers. According to Gild, frequenting a particular Japanese manga site is a “solid predictor of strong coding” — despite the fact that women have less leisure time to spend online than men and often don’t like manga sites, which are dominated by men.”
Similarly, last year Amazon shut down an AI recruiting tool for showing bias against women.
We all have blind spots and are deluding ourselves to suggest otherwise. The above articles got me thinking about Indeed and where our blind spot could come from. A few ideas:
College Degrees — According to the Census Bureau, about one-third of Americans 25 or older have a bachelor’s degree. This number probably rounds to 100% for most product and engineering teams.
Household Income — Median household income in the US is $63k. Median household income in Seattle — a tech heavy city — is $93k. The median salary for a software engineer in the US is $106k. This compares to $60k for a truck driver, $30k for a hair stylist, $27k for a customer service representative, and $25k for a retail sales associate.
Access to Technology — While computer and smartphone access is something we take for granted, about 10% of US households don’t have a computer and roughly 20% don’t have a smartphone, per the Census Bureau.
The situation at Uber was something like this:
Uber’s engineering team is male dominated → Issues like personal safety and sexual assault were not top of mind → The team had a blind spot around safety → 3,000 cases of sexual assault were reported during Uber rides last year
The parallel for Indeed might look like this:
Our engineering teams are generally composed of well educated, well paid employees → Relative to our engineering teams (and Indeed in general), our job seekers tend to have less education and lower salaries → The job search experience of our job seekers is likely to be different from the job search experience of the people building products for our job seekers
Not sure what the consequence of this is, but you can see how there’s room for error and missed opportunities.
Every person and every organization has blind spots. With things slowing down into year end, it’s worthwhile to spend time reflecting on what these could be.